Markov chain mode - Study guides, Class notes & Summaries
Looking for the best study guides, study notes and summaries about Markov chain mode? On this page you'll find 3 study documents about Markov chain mode.
All 3 results
Sort by
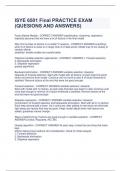
-
ISYE 6501 Final PRACTICE EXAM (QUESIONS AND ANSWERS)
- Exam (elaborations) • 11 pages • 2024
- Available in package deal
-
- $13.49
- + learn more
ISYE 6501 Final PRACTICE EXAM 
(QUESIONS AND ANSWERS) 
Factor Based Models - CORRECT ANSWER-classification, clustering, regression. 
Implicitly assumed that we have a lot of factors in the final model 
Why limit number of factors in a model? 2 reasons - CORRECT ANSWER-overfitting: 
when # of factors is close to or larger than # of data points. Model may fit too closely to 
random effects 
simplicity: simple models are usually better 
Classical variable selection approaches - CORRECT ANSWER-1....
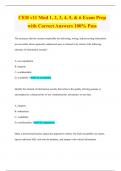
-
CEH v11 Mod 1, 2, 3, 4, 5, & 6 Exam Prep with Correct Answers 100% Pass
- Exam (elaborations) • 151 pages • 2023
-
- $14.99
- + learn more
CEH v11 Mod 1, 2, 3, 4, 5, & 6 Exam Prep with Correct Answers 100% Pass 
 
The assurance that the systems responsible for delivering, storing, and processing information are accessible when required by authorized users is referred to by which of the following elements of information security? 
 
A. non-repudiation 
B. integrity 
C. confidentiality 
D. availability *ANS* D. availability 
 
Identify the element of information security that refers to the quality of being genuine or uncorrupted as a...
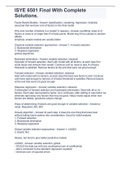
-
ISYE 6501 Final With Complete Solutions.
- Exam (elaborations) • 10 pages • 2022
-
- $11.99
- + learn more
Factor Based Models 
classification, clustering, regression. Implicitly assumed that we have a lot of factors in the final model 
 
 
 
Why limit number of factors in a model? 2 reasons 
overfitting: when # of factors is close to or larger than # of data points. Model may fit too closely to random effects 
 
simplicity: simple models are usually better 
 
 
 
 
Classical variable selection approaches 
1. Forward selection 
 
2. Backwards elimination 
 
3. Stepwise regression 
 
greedy algorithms...
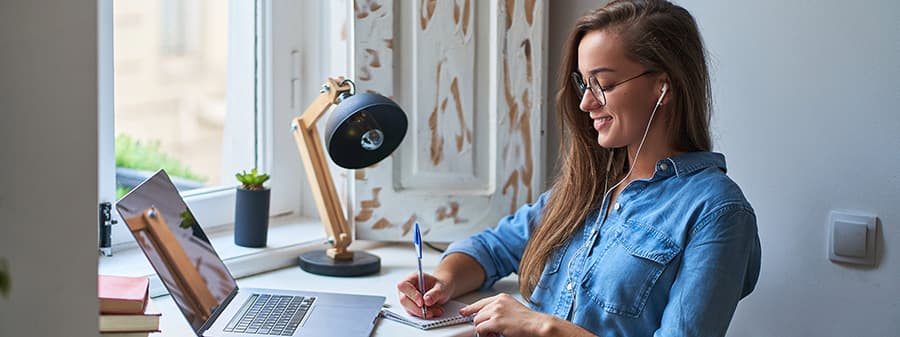
Study stress? For sellers on Stuvia, these are actually golden times. KA-CHING! Earn from your study resources too and start uploading now. Discover all about earning on Stuvia